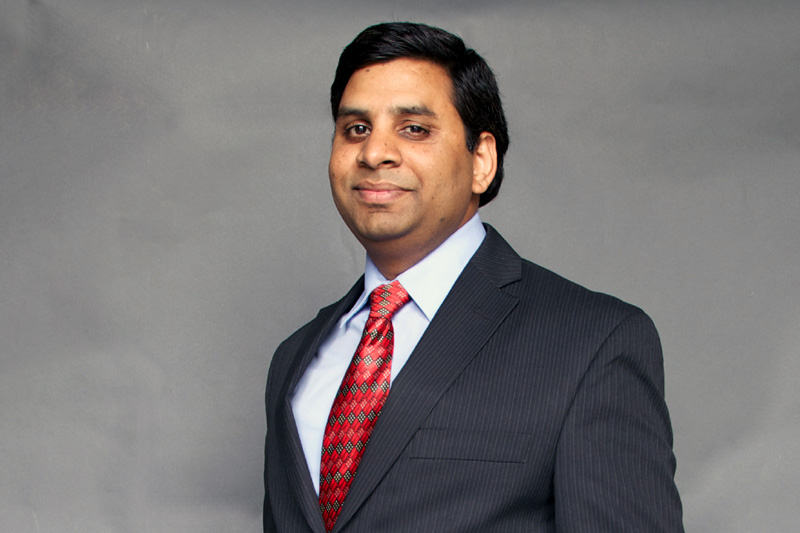
A cross-disciplinary research initiated by Prof. Chandra Singh and his collaborators, Gurjot Dhaliwal (PhD. Candidate, MIE) and Prof. Prasanth B. Nair (Professor, UTIAS), has led to the discovery of novel and computationally efficient methods for interatomic interactions to be used in molecular dynamics simulations.
“Computational materials science has led to significant discoveries of new materials and their property predictions.” says Prof. Singh. “It is known as the third paradigm for materials development, following the experimental and theoretical materials science. More recently, machine learning has emerged as the fourth paradigm for materials discovery.”
As computer simulations of materials at atomic scale are very cumbersome, Prof. Singh and his collaborators are working together on leveraging machine to develop more computationally-efficient models to accelerate these atomistic simulations. In their latest study, published in Nature Partner Journal, Computational Materials, they present a method to model interatomic potentials that are at the heart of atomic simulations, using machine learning techniques.
“Apart from generalization (towards different material classes) and accuracy, these potentials are also computationally efficient than other existing machine learning based approaches,” says Dhaliwal, Singh’s PhD student. “The linear nature of these potentials helps in efficient parameter estimation than neural networks and kernel-based models.”
Read the full article