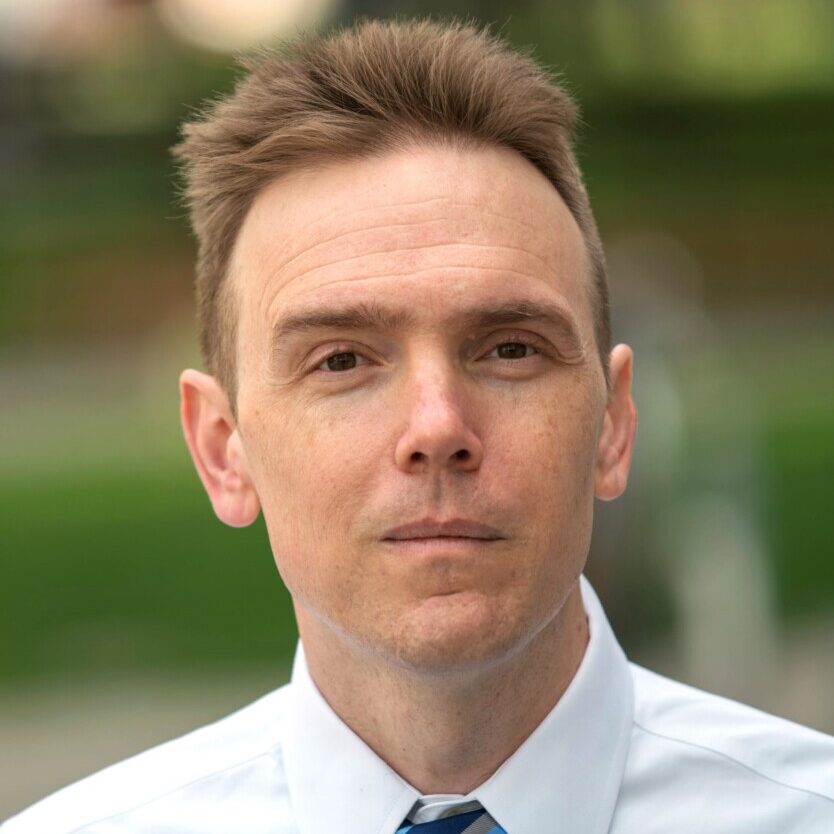
Jason Hattrick-Simpers | BSc (Rowan), PhD (Maryland)
Professor
Research Group: AUTOnomous DIscovery of ALloys (AUTODIAL)
E: jason.hattrick.simpers@utoronto.ca
Office: WB156
Research Areas:
We Discover Novel Corrosion Resistant Compositionally Complex Alloy Coatings
Prevention and remediation of environmental degradation through corrosion has been estimated to cost Canada $41 billion per year and there is a constant need for new materials that can extend the lifetime of vehicles, pipelines, airplanes, nuclear cladding materials, buildings, and bridges. We use automated experimental platforms and AI to explore the role of composition, processing, and environment on the performance of high entropy alloys, metallic glasses, intermetallics, and ceramic coatings. We then work with collaborators at industrial and national labs around the world to scale up our discoveries with the goal of bringing them market.
We Develop Autonomous Materials Science Tools
The intersection of experimental automation, artificial intelligence, and materials data infrastructure represents an exciting opportunity to substantially accelerate the identification of novel materials (potentially up to 1,000x). Ideally these tools have the power to initialize new experiments, plan their execution, conduct the experiment, analyze the data, and draw conclusions about the outcomes. The melding of these tasks erodes the traditional segmentation of synthesis and characterization tasks and gives us the exciting opportunity to rethink the design of the very tools we use as materials scientists. As a part of our work, we develop and apply integrated closed-loop platforms that enable the validation of AI-human generated hypotheses at the rate of generation.
We Develop and Test Scientific AIs to Increase Trust and Adoption in the Physical Sciences
AI and traditional scientific modeling are often discussed as distinct and opposite paradigms. But really, they are complementary to one another: the former extrapolates poorly but can flexibly fit any (sufficiently large) dataset while the latter can extrapolate well within its range of applicability but is brittle outside that range. We work with our collaborators in Canada and abroad to develop scientific-AIs where scientific rules and heuristics are directly built into AIs creating algorithms that leverage the strengths of both approaches. The goal is to use scientific AI to not only optimize existing materials and discover new materials, but also to further our mechanistic understanding of materials and how they behave.
We Democratize Science by Sharing Data, Scientific Code, and Developing Open and Inexpensive Tools
As the sophistication (and cost) of scientific tools has increased a chasm has emerged between those who are empowered to do science and those who are not, effectively pricing out the citizen scientist. This limits diversity and representation in science, increases science skepticism among the general public, and contributes to the replication crisis in science. Inspired by the open source and maker communities, we emphasize open scientific inquiry by creating combined synthesis and characterization tools that can be recreated by anyone in the world; likewise, all schematics, automation protocols, scientific data and code generated via our lab is made available to the public via our Github page.
Research Cluster(s)
Jason Hattrick-Simpers is a Professor at the Department of Materials Science and Engineering, University of Toronto and a Research Scientist at CanmetMATERIALS. He graduated with a B.S. in Mathematics and a B.S. in Physics from Rowan University and a Ph.D. in Materials Science and Engineering from the University of Maryland. Prof. Hattrick-Simpers’s research interests focus on the use of AI and experimental automation to discover new functional alloys and oxides that can survive in extreme environments and materials for energy conversion and storage. Specific topics of interest to the group include corrosion resistant ultra-hard alloys, oxides, nitrides, and carbides; thermoelectric materials for heat to energy conversion; novel metals for hydrogen fueling stations; and oxides for CO2 conversion.
Prior to joining UofT Prof. Hattrick-Simpers was a staff scientist at the National Institute of Standards and Technology (NIST) in Gaithersburg, MD where he co-developed tools for discovering novel corrosion resistance of alloys, developed active learning approaches to guide thin film and additive manufacturing alloy studies, and developed tools and best practices to enable trust in AI within the materials science community. He has published over 80 papers and given more than 50 invited seminars and talks. He was an associate editor of ACS Combinatorial Science from 2017 – 2020 and is part of the organizing committee for the International Workshop on Combinatorial Materials Science and Technology.
Link to Google Scholar
Select Publications:
Book Chapters and Blog Posts
- Hattrick-Simpers, J.R., “How Robots Could Teach Us to Trust AI” a. Highlighted by Medium.com (https://medium.com/@nist/how-robots-could-teach-us-to-trust-ai-d1d0db5a51ed)
- Green, M.L., Joress, H., Takeuchi, I., Hattrick-Simpers, J.R.; “Applications of High Throughput (Combinatorial) Methodologies to Electronic, Magnetic, Structural, and Energy-Related Materials” Encyclopedia of Materials: Metals and Alloys (2021)
Recent Journals:
- DeCost, B. L., Hattrick-Simpers, J.R., Trautt, Z., Kusne, A.G., Campo, E., Green, M.L.; “Scientific AI in materials science: a path to a sustainable and scalable paradigm” Machine Learning: Science and Technology, Vol 1, no. 3 03301 (2020)
- Hattrick-Simpers, J.R., Zakutayev, A., Barron, S.C., Trautt, Z.T., Nguyen, N., Choudhary, K., DeCost, B., Phillips, C., Kusne, A.G., Yi, F., Mehta, A., Takeuchi, I., Perkins, J.D., Green, M.L.; “An inter-laboratory study of Zn-Sn-Ti-O thin films using high-throughput experimental methods” ACS Combinatorial Science Vol. 21, no. 5 pp 350-361 (2019)
- Vasudevan, R.K., Choudhary, K., Mehta, A., Smith, R., Kusne, A.G., Francesca, T., Vlcek, L., Ziatdinov, M., Kalinin, S.V., Hattrick-Simpers, J.R.; “Materials science in the AI age: high-throughput library generation, machine learning and a pathway from correlations to the underpinning physics” Accepted to MRS Communications (April 2019)
- Meredig, B., Antono, E., Church, C., Hutchinson, M., Ling, J., Paradiso, S., Blaiszik, B., Foster, I., Gibbons, B., Hattrick-Simpers, J.R., Mehta, A., Ward, L.; “Can machine learning identify the next high-temperature superconductor? Examining extrapolation performance for materials discovery” Molecular Systems Design and Engineering (2018)
- Ren, F., Ward, L., Williams, T., Laws, K.J., Wolverton, C.W., Hattrick-Simpers, J.R., Mehta, A.; “Accelerated discovery of metallic glasses through iteration of machine learning and High-Throughput Experiments” Science Advances Vol 4, no. 4 (2018)
- Green, M.L., Choi, C.L., Hattrick-Simpers, J.R., Joshi, A.M., Takeuchi, I., Barron, S.C., Campo, E., Chiang, T., Empedocles, S., Gregoire, J.M., Kusne, A.G., Martin, J., Mehta, A., Persson, K., Trautt, Z., Van Duren, J. Zakutayev, A.; “Fulfilling the promise of the materials genome initiative with high-throughput experimental methodologies” Applied Physics Reviews Vol. 4 no. 1 011105 (2017)
- Ruiz-Yi, B., Bunn, J.K., Stasak, D., Mehta, A., Besser, M., Kramer, M.J., Takeuchi, I., Hattrick-Simpers, J.R. “The different roles of entropy and solubility in high entropy alloy stability” ACS Combinatorial Science Vol. 18, no. 3, pp 596-603 (2016)
- Hattrick-Simpers, J.R., Gregoire, J., Kusne, A.G.; “Composition – structure – property mapping in high-throughput experiments: turning data into knowledge”, Invited article APL: Materials Vol. 4 053211 (2016)
- Bunn, J.K., Fang, R.L., Albing, M.R., Mehta, A., Kramer, M.J., Besser, M.F., Hattrick-Simpers, J.R.; “A high-throughput investigation of Fe-Cr-Al as a novel high-temperature coating for nuclear cladding materials” Nanotechnology Vol. 26 no. 27 page 274003 (2015)
- Bunn, J.K., Metting, C.J., Hattrick-Simpers, J.R.; “A semi-empirical model for tilted-gun planar magnetron sputtering accounting for chimney shadowing” JOM Society special issue on Integrated Computational Materials Engineering Vol. 65 pp. 154 – 163 (2015)
MSE465/1065: Application of Artificial Intelligence in Materials Design
MSE403: Data Sciences and Analytics for Materials Engineers